DeepFE: Deep learning for frog eggs quantification
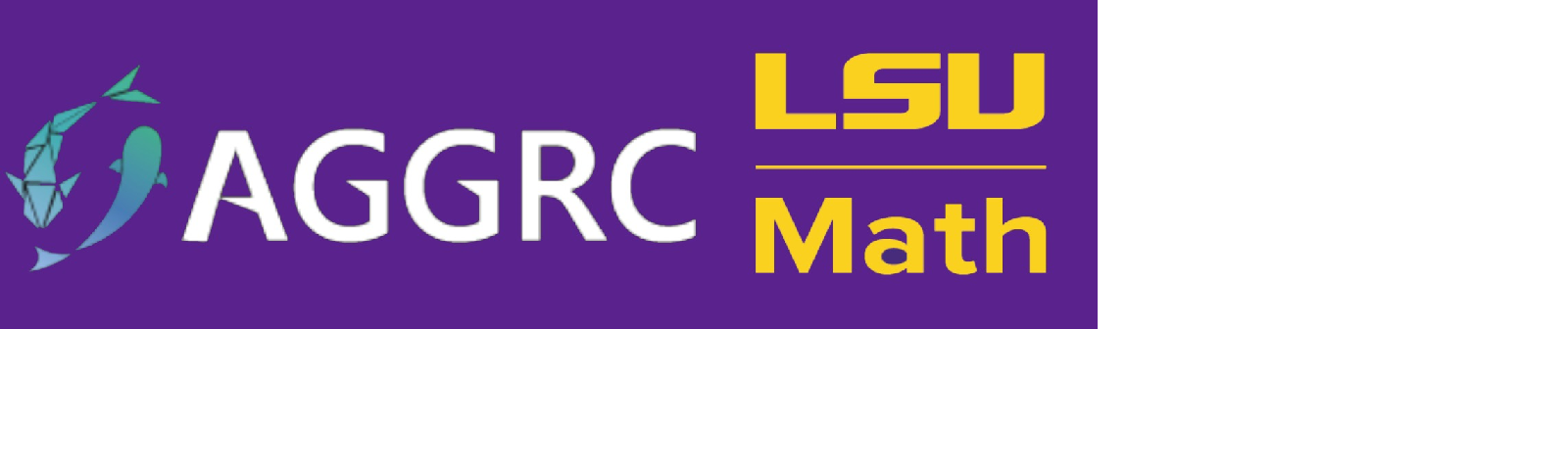
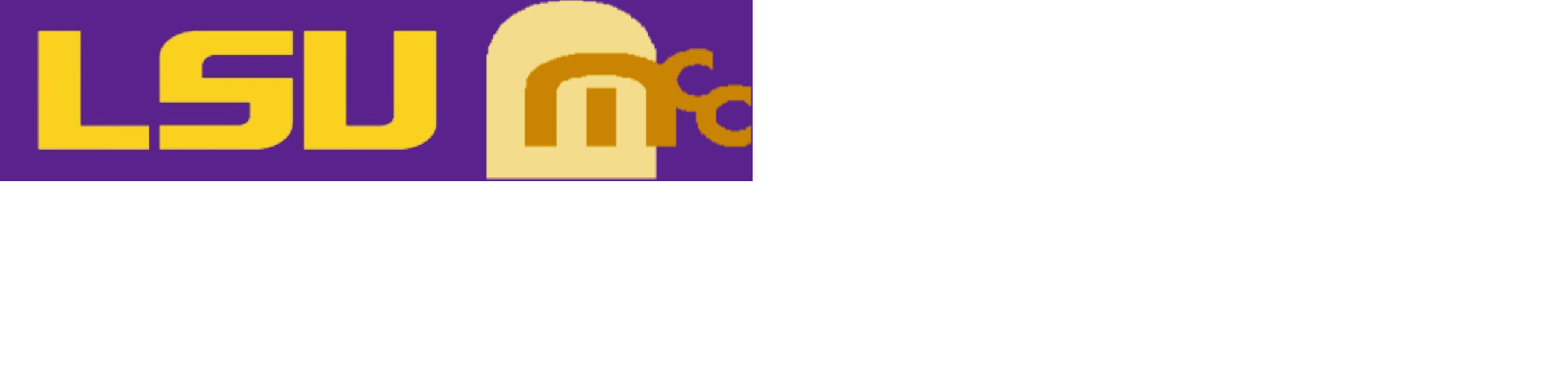
The project's goal is to count frog eggs in a Petri dish. AGGRC asked for MC^2's help to tackle their main challenge: accurately counting frog eggs, especially in a clustered environment, as the existing methods were not performing well in such cases. MC^2 took on the task and developed a program to provide AGGRC with a better and faster egg counting solution, suitable for both clustered and non-clustered eggs. Throughout the summer of 2023, the team focused on building a machine-learning model specifically for non-clustered eggs, aiming to surpass the accuracy and speed of AGGRC's current tools. The new model is based on the Stardist machine learning package, which uses image segmentation and star-convex polygons to count eggs by identifying cell nuclei. To evaluate the model, they conducted experiments using a dataset of frog eggs, achieving an impressive accuracy of over 90 percent during the training process, using 180 images over 150 epochs. Creating the right dataset involved careful preprocessing, done with the help of the FIJI application and lab kit plugins. Each egg was annotated to establish the total egg count, ensuring the model's training data was of good quality.